Understanding the True Value of Data Analytics in 2024
Written on
Chapter 1: The Importance of Time in Analytics
The initial phase of discussing analytics at conferences often involves persuading the audience of its merits. However, the tone shifts dramatically when I reveal the underlying truth: engaging in analytics requires a significant investment of time. Just like any investment, there is a possibility of not reaping any rewards.
Analytics demands a substantial commitment of time, and similar to other investments, it carries the risk of yielding no returns. For a culture of analytics to flourish, organizations must foster environments where analysts are not penalized for coming back without tangible results.
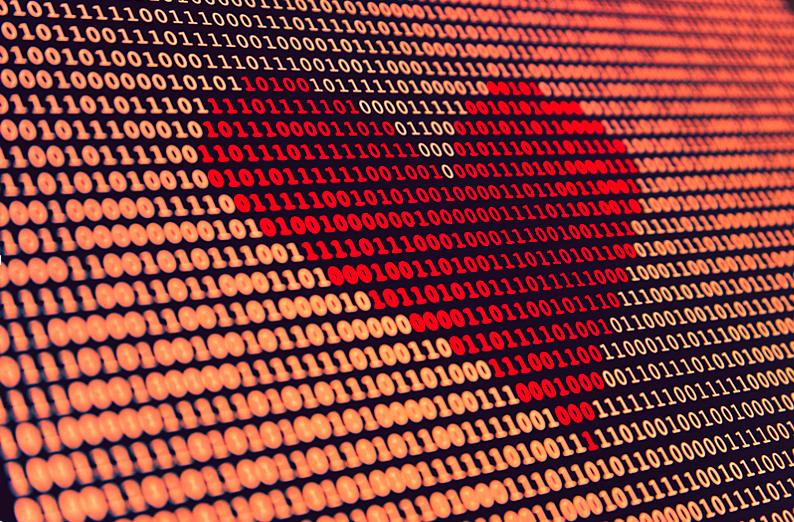
This article continues a series that highlights ten key distinctions between amateur and professional analysts. The first eight differences were elaborated upon in previous articles, and now we delve deeper into the nuances of professional analysis.
Section 1.1: Differentiating Between Types of Analytics
Data analytics can be categorized into two distinct types:
- Reactive Analytics: This involves retrieving data to address specific queries posed by the team.
- Proactive Analytics: This entails examining data to spark new ideas and directions within the team.
The most impactful role an analyst can fulfill is to motivate decision-makers to contemplate options they may not have previously considered. This process, known as exploratory data analysis (EDA), is integral to proactive analytics.
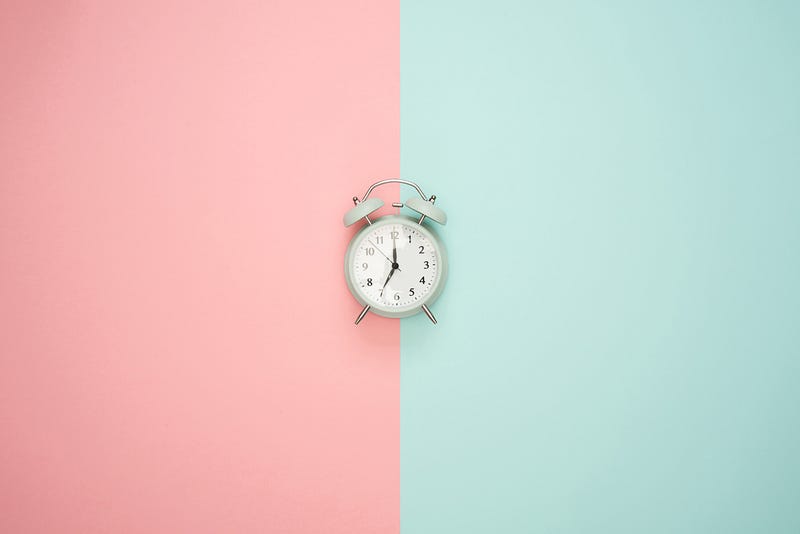
Now, brace yourself for an uncomfortable reality: this crucial contribution of an analyst often involves a time investment without guaranteed outcomes. Experienced analysts gravitate towards leaders who share a correct understanding of analytics.
Professional analysts recognize this challenging reality and actively seek out leaders who are willing to make irreversible investments and accept responsibility for them. Unfortunately, such leaders are scarce, and organizations that cultivate an environment for analysts to thrive are even rarer. Establishing a culture of analytics that comes with no strings attached requires considerable courage.
Chapter 2: Professional Mindset Towards Time
The first video, "A Day in the Life of a Data Analyst (2023)," provides insights into the daily responsibilities of a data analyst, emphasizing the importance of time management and analytics.
Professional analysts grasp that their work is not about guaranteeing outcomes but rather about investing time into inspiration, innovation, and early detection of potential issues.
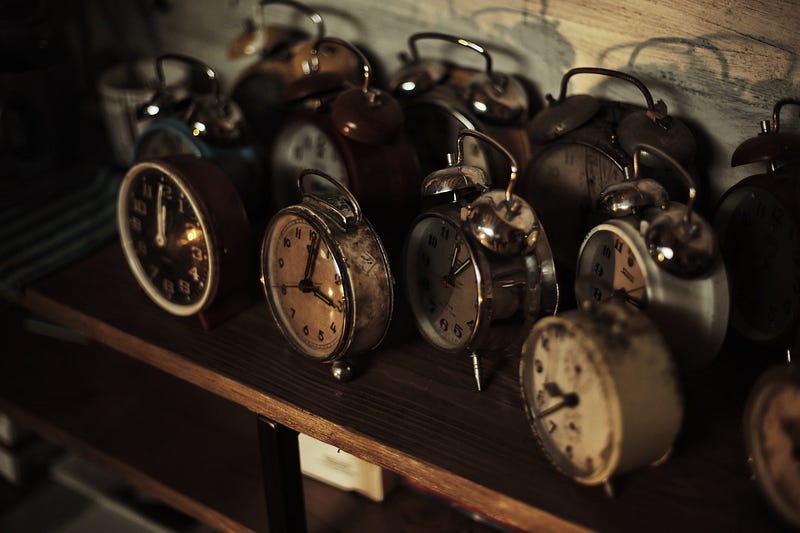
In an adept organization, analysts are trusted to utilize their allotted time effectively. They prioritize understanding the return on investment (ROI) of their efforts, employing the following approaches:
- Systematic Work: Focusing on the most promising inquiries and being vigilant for signs of dead ends to pivot early.
- Iterative Processes: Starting with the broader context and gradually honing in on specific details while maintaining communication with decision-makers.
- Quick Execution: Utilizing a coding style that maximizes inspiration and creativity, which may differ from traditional production-ready code.
- Smart Investment: Allocating sufficient time to understand data quality, ensuring that their efforts are not wasted on ineffective data sources.
- Holistic Approach: Integrating insights from diverse data sources, including documentation and stakeholder discussions.
- Proactive Engagement: Anticipating questions from decision-makers and suggesting new data avenues for exploration.
- Strategic Focus: Recognizing that their time investment is a resource from decision-makers and striving to maximize overall ROI.
Few roles prioritize time ROI as intensely as that of a data analyst. Adopting a nuanced perspective on speed is critical for achieving excellence in the field and advancing in one’s career.
The second video, "Step by Step Process of Thinking Like a Data Analyst," offers a comprehensive guide on cultivating the right mindset and methodologies for aspiring analysts.
This concludes our exploration of the differences between amateur and professional analysts, focusing on the vital role that time plays in analytics.