Will AI Surpass Human Intelligence? Insights into Surrogate Losses
Written on
Chapter 1: Understanding Surrogate Losses
Imagine predicting events before they unfold, such as weather changes or sports outcomes. In machine learning, researchers develop models that facilitate these predictions. A key element in this process is known as "surrogate losses." These specialized functions assist in training models when the actual prediction tasks are too complex to handle directly.
Surrogate Losses: The Unseen Helpers
Surrogate losses function similarly to training wheels on a bicycle—they streamline the learning experience. In both binary and multi-class classifications, which categorize data into two or more groups, these losses simplify intricate data relationships. This simplification allows computers to learn effectively and make accurate predictions based on historical data.
Exploring Multi-Class Classification
As we delve deeper into machine learning, we come across multi-class classification, which deals with more intricate decision-making scenarios. For example, instead of merely identifying whether an image is of a cat or a dog, we could also include other animals like lions, tigers, and bears! Surrogate losses enable us to navigate this complexity by offering a structure to manage multiple categories seamlessly.
Growth Rates and Efficiency of Learning
A crucial insight regarding surrogate losses is their growth rate within learning algorithms. This metric indicates how swiftly a learning model can enhance its accuracy. Recent research has revealed that specific types of surrogate losses exhibit a universal "square-root" growth rate, providing a reliable framework for gauging how improvements in learning methods translate into better performance in real-world applications.
Surrogate Losses in Neural Networks
In neural networks—sophisticated models that mimic the human brain—surrogate losses prove particularly effective. They empower these networks to learn from extensive data sets without becoming overwhelmed by complexity. This capability is essential for tasks like voice recognition and language translation, where capturing the subtleties of human communication is vital.
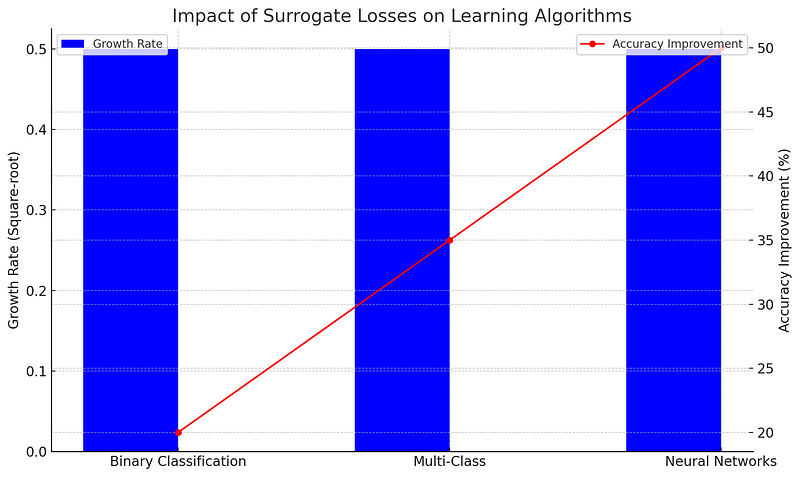
The graph illustrates the consistent support that surrogate losses provide across various models, showcasing significant enhancements in prediction accuracy, especially in intricate applications like neural networks.
Universal Relevance of Surrogate Losses
Surrogate losses are not confined to a single type of learning model; they are applicable across diverse machine learning algorithms. This versatility renders them invaluable in the realm of artificial intelligence.
Accelerating the Learning Process
By simplifying the initial learning challenges, surrogate losses can greatly expedite the learning process. This acceleration is crucial for applications requiring real-time decision-making, such as in self-driving cars.
Enhancing Prediction Accuracy
When surrogate losses are optimized, they contribute to improved prediction accuracy. This enhancement occurs because these losses aid in effectively fine-tuning the parameters of learning models.
Addressing Non-Differentiable Challenges
Many real-world scenarios present non-differentiable problems, which traditional mathematical tools struggle to solve. Surrogate losses enable learning algorithms to tackle these issues by approximating them in a differentiable manner.
Facilitating Complex Classifications
In multi-class classification contexts, surrogate losses help to navigate complexity by deconstructing the problem into simpler components. This strategy ensures that the learning algorithm remains both efficient and effective, even as task complexity escalates.
A Promising Future with Surrogate Losses
Exploring the realm of surrogate losses reveals a future brimming with potential. These tools not only enhance learning algorithms' capabilities but also pave the way for innovations that could revolutionize our daily lives. From advanced healthcare diagnostics to more intuitive technology interfaces, the opportunities are limitless. As we continue to refine these methods, we can anticipate a future where technology comprehends and supports us in increasingly sophisticated manners, enhancing our connectivity and ease of living.
Chapter 2: The Future of AI and Robotics
In this TEDx talk, Mateja Jamnik explores the question: will AI surpass human intelligence? The discussion delves into the implications of advanced AI systems and their potential roles in society.
Ben Goertzel discusses when we might see robots taking over significant roles in our lives, examining the trajectory of AI advancements and their societal impact.
About Disruptive Concepts
Welcome to @Disruptive Concepts—your insight into the future of technology. Subscribe for new videos every Saturday!
Watch us on YouTube.