The Growing Need for Specialization in AI & ML Engineering
Written on
Chapter 1: The Evolution of AI Roles
Technology is characterized by constant change, and this is particularly evident in the fields of Artificial Intelligence (AI) and Machine Learning (ML). Over the last ten years, significant advancements in tools and their abstraction have made it easier for various organizations to develop AI-powered software solutions. This shift has not only reduced entry barriers for many in the software industry but has also ignited transformative changes in how we approach AI development.
This leads to a thought-provoking question for industry leaders: Can we anticipate the landscape of AI roles in the next five years?
To explore this, we will examine how technological advancements catalyze changes in roles related to AI, starting with one of the foundational positions in AI development: The Data Scientist.
Section 1.1: Tracing the Data Scientist’s Journey
To better predict the future, we should reflect on the past. Let’s go back to 2010, a pivotal year marked by significant events such as the Deepwater Horizon disaster and the launch of Instagram. During this time, data scientist Drew Conway introduced his now-famous “Data Science Venn Diagram.”
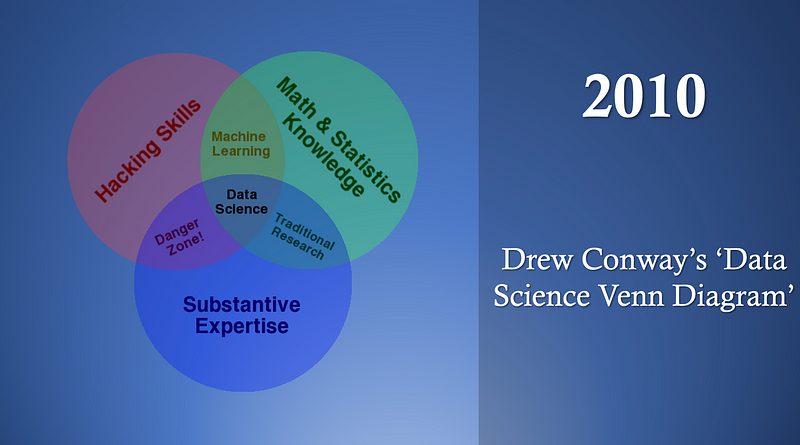
Conway envisioned the data scientist role as a blend of programming skills, statistical knowledge, and experience, capturing the essence of the classical data scientist at the decade's start. However, as we know, the technology landscape is ever-evolving, and roles must adapt accordingly.
Now, let’s fast forward to 2014, a year that saw the Brazil World Cup and the Flint water crisis in the USA.
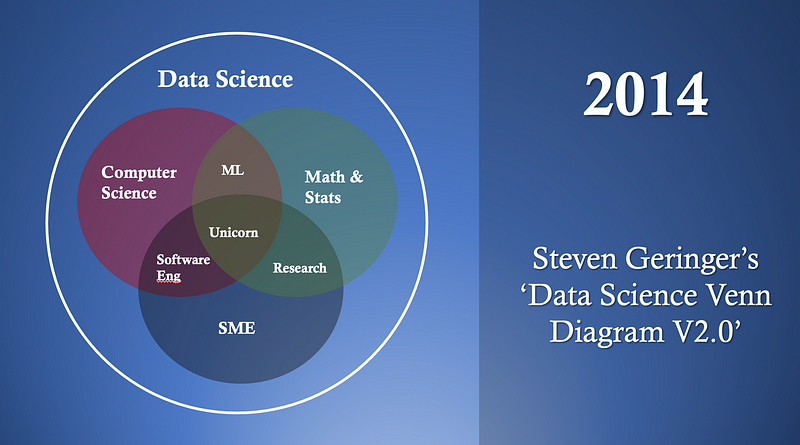
During this time, Stephen Geringer expanded the Venn diagram concept, suggesting that various skills—ranging from computer science to subject matter expertise—now fit within the broader field of data science. His premise was simple: if you handle data in any capacity, you belong to the realm of data science.
This idea highlights that data science not only encompasses more specialized roles but also relies on a diverse skill set to function effectively.
Subsection 1.1.1: The 2017 Shift
Now let’s return to our time machine and land in 2017, when the UK initiated the “Article 47” Brexit protocol.
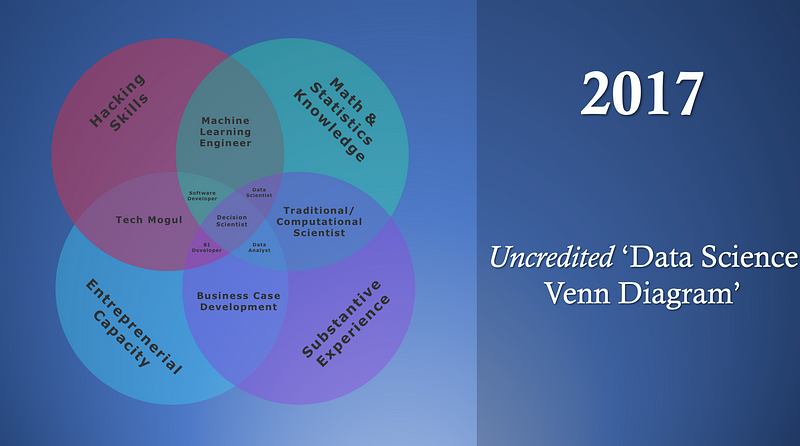
By this point, organizations began to clearly differentiate between various data roles, with data analysts, BI engineers, and decision scientists emerging as distinct specializations from the traditional data scientist role.
Chapter 2: The Splintering of AI Engineering Roles
As an observer of technical changes within the AI landscape, it’s clear that the AI engineer role is evolving, requiring additional specializations.
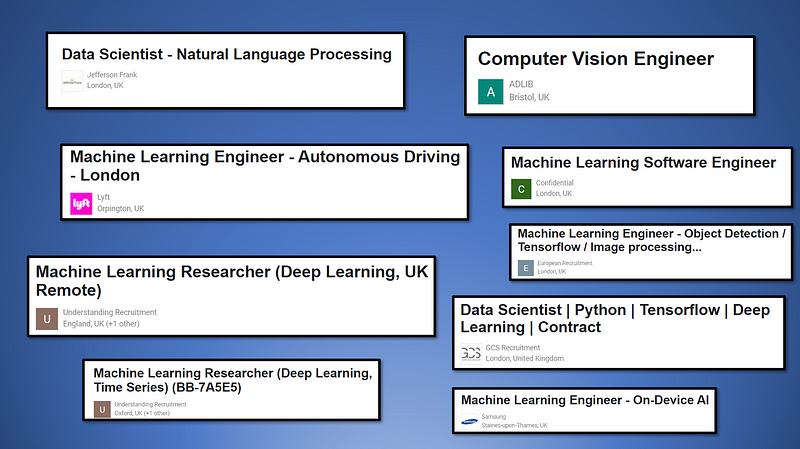
AI encompasses a wide array of expertise, making it nearly impossible for one individual to master every aspect thoroughly. This reality is a significant factor driving the demand for specialized roles within the AI domain.
With the AI engineer position undergoing fragmentation, can we look to the development of other technologies for insights into how we might shape our AI capabilities moving forward?
Section 2.1: Drawing Parallels with JavaScript
Lessons can be gleaned from the dynamic evolution of JavaScript frameworks, which offers intriguing similarities to the contemporary development of AI tools and roles.
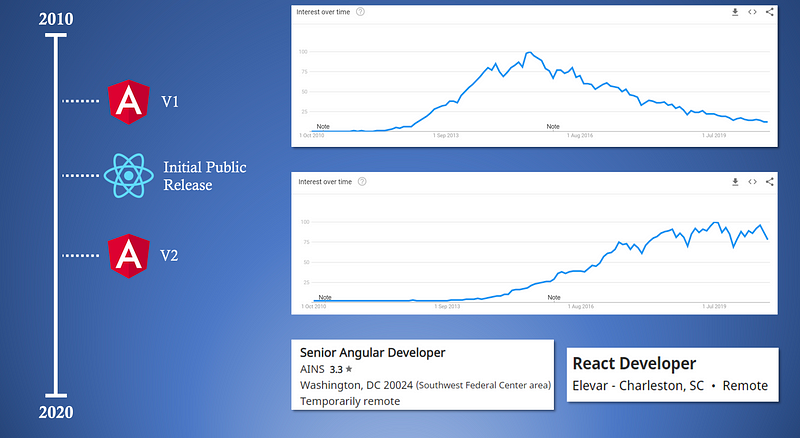
AngularJS and React were both released around the start of the last decade. A look at Google Trends reveals that AngularJS enjoyed a surge in popularity following its launch but saw a decline as React gained traction, illustrating the ever-changing landscape of JavaScript tools.
This rapid evolution has made it challenging for JavaScript developers to remain generalists, leading to a rise in role specialization, particularly for Angular and React developers.
Subsection 2.1.1: AI Frameworks in Comparison
A similar analysis can be applied to two popular Python AI frameworks—TensorFlow and PyTorch.
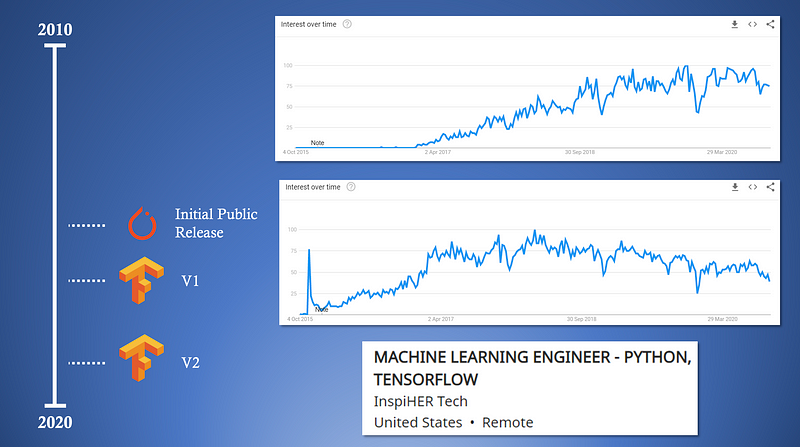
TensorFlow, despite being an early entrant, has seen its popularity plateau, while PyTorch continues to rise. PyTorch’s user-friendly API is one reason for its popularity, while TensorFlow struggled until the release of TensorFlow 2.
As highlighted throughout this discussion, we see a consistent outcome: the push toward specialization.
Chapter 3: Insights from Technology Trends
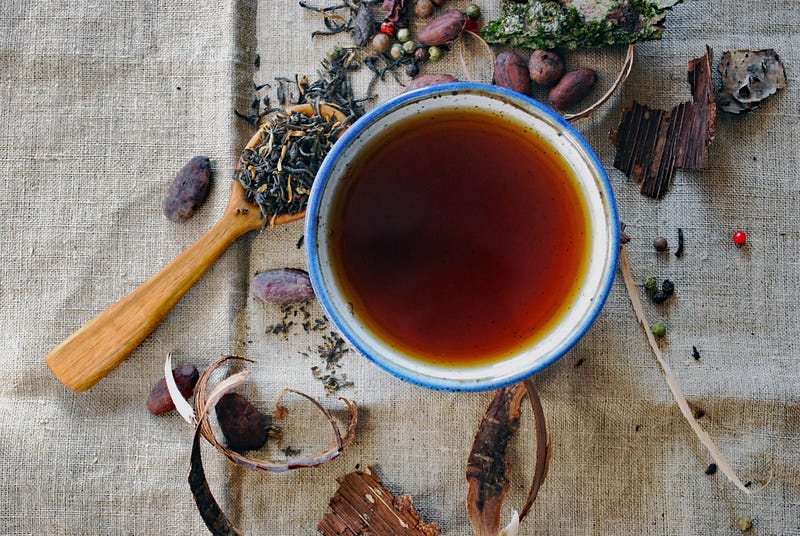
Current trends suggest that AI development is mirroring the path taken by JavaScript, albeit with a delay of around five years. Can we leverage historical insights from JavaScript to plan for the future of AI? Will AI follow a familiar trajectory, or will it forge a unique path? These are crucial questions for leaders to consider as they strategize around AI technologies.
In my view, AI is likely to closely follow the evolution of JavaScript, resulting in increased specialization for existing tools and the emergence of critical advancements in MLOps, which is gaining traction as a vital component of any AI solution.
Conclusion
In conclusion, we have explored several key points:
- The transition of the classical data scientist role to its modern counterpart.
- The parallels between the evolution of AI and JavaScript.
- Speculations about the future of AI engineering roles and the drive for increased specialization.
The fundamental takeaway is that to predict the future, one must first understand the past. AI does not exist in isolation; a broader perspective on software trends is essential for effective AI strategy planning.
This article serves as part one in a series of blogs. A follow-up piece will delve into whether the trend of role specialization in AI poses challenges for organizations.