Apache Spark and Flink: Choosing the Right Tool for Your Needs
Written on
Chapter 1: Introduction to Spark and Flink
Apache Spark and Apache Flink have emerged as significant players in the realm of big data processing. Each framework brings a robust set of features tailored for extensive data handling and real-time analytics. Nevertheless, their unique characteristics cater to different scenarios, making it essential to understand their distinctions. In this discussion, we will explore the critical differences between Spark and Flink, helping you identify the best option for your data processing tasks.
Section 1.1: Processing Models
Spark:
- Capable of managing both batch and real-time processing.
- Optimized for large-scale data operations with a high-performance execution engine.
Flink:
- Prioritizes stream processing as its core function.
- Integrates batch and stream analytics seamlessly, offering a unified API for both continuous and batch data handling.
Section 1.2: Fault Tolerance Mechanisms
Spark:
- Achieves fault tolerance via Resilient Distributed Datasets (RDDs), enabling recovery of lost data partitions and ongoing processing during failures.
Flink:
- Utilizes a distributed snapshot approach for fault tolerance, ensuring consistent results by restoring the system's state following failures.
Chapter 2: Event Time Processing and State Management
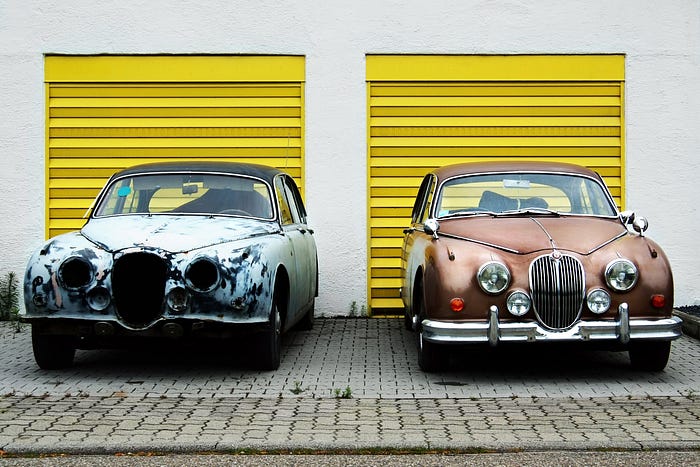
Event Time Processing:
Spark:
- Provides event time processing, albeit with certain constraints, necessitating explicit management of watermarks and event time skew.
Flink:
- Excels in event time processing, particularly suited for real-time analytics. It simplifies the management of out-of-order events and supports advanced time-based aggregations.
State Management:
Spark:
- Relies on external storage systems, such as Hadoop Distributed File System (HDFS), for state management, which introduces potential network overhead.
Flink:
- Maintains state internally, streamlining stateful processing and enhancing performance by reducing data movement across networks.
Chapter 3: Ecosystem and Connectivity
Spark:
- Highly adopted with a vast ecosystem, integrating seamlessly with various data sources, connectors, and libraries. It is well-aligned with popular big data tools like Apache Hadoop, Hive, and Kafka.
Flink:
- Boasting a rapidly expanding ecosystem focused on event-driven applications, it offers robust connectors for diverse data streams, particularly appealing for event-driven architectures.
Section 3.1: Data Processing Latency
Spark:
- Best suited for applications with moderate to high latency demands. It delivers excellent performance for batch processing and reasonable latency for real-time analytics.
Flink:
- Specifically designed for low-latency applications, achieving processing delays in the millisecond range, making it ideal for real-time scenarios.
Conclusion
In summary, both Apache Spark and Apache Flink are formidable tools in the landscape of big data processing and real-time analytics. Spark's adaptability, established ecosystem, and dual support for batch and real-time processing render it a strong candidate for various applications. Conversely, Flink's emphasis on stream processing, effective event time handling, internal state management, and low-latency capabilities position it as the preferred choice for real-time applications demanding stringent latency requirements. Evaluating your specific needs regarding processing models, fault tolerance, event time handling, and latency expectations will aid you in selecting the most appropriate framework for your data challenges.
Explore the differences between Apache Spark and Apache Flink from the perspective of various companies in this insightful video.
This comparison video delves into the features and functionalities of Apache Spark and Flink as big data processing tools.